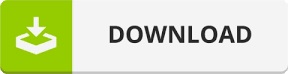
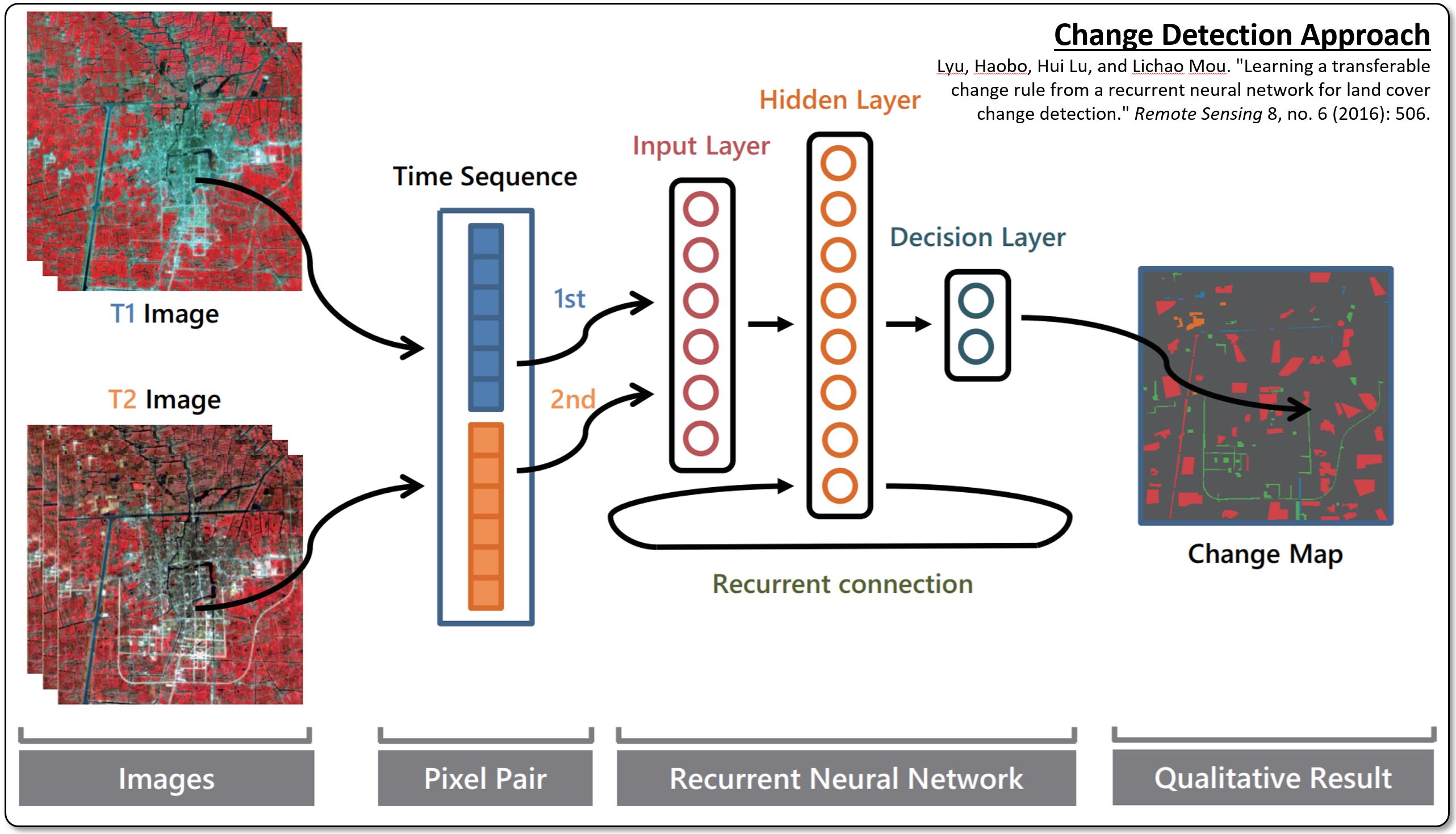
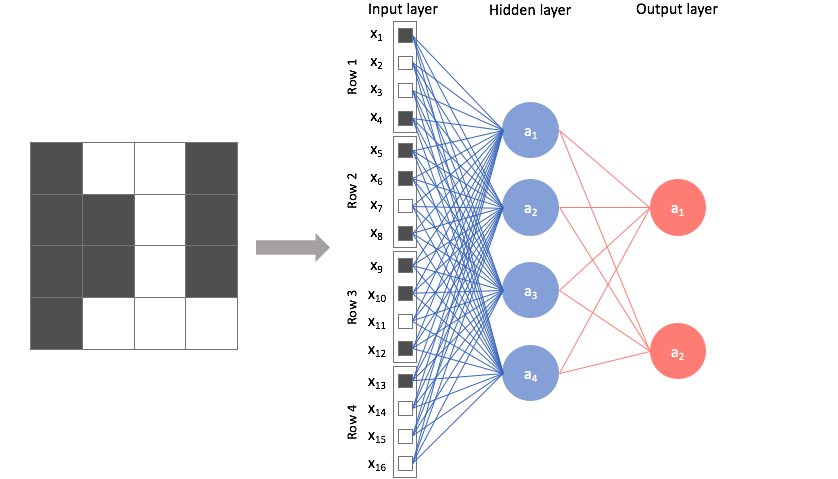
NEURAL NETWORK RADAR SERIES
Computationally efficient and scalable approach for classification of hydrometeors offer opportunties to effectively use the large volumes of rich time series of radar observations that are becoming increasingly available. Seasonal variation in atmospheric conditions lead to seasonal more » patterns of liquid vs solid forms of precipitation, that poses challenge for classifier and offer insights into domain specific approaches required for problem of hydrometeor identification. Training data selection strategies were important to ensure use of available samples in training for robust performance evaluated by applying the models to novel time period beyond what was use to train the model. We developed and optimized five Convolutional Neural Networks (CNNs) architectures and achieved an accuracy of 87.23% and 93.736% respectively using modified ResNet with two different input data selection strategies for hydrometeor classification. Samples are preprocessed and divided into training, validation and test set with four target hydrometeor categories (Ice Crystals (IC), Dry Snow (DS), Light and/or Moderate Rain (RA) and Big Drops (rain) (BD)). We stack matrices of values from these four polarimetric variables as one 3D array. We propose a deep learning-based method to estimate hydrometeors efficiently using observed radar variables such as horizontal reflectivity (Z H ), differential reflectivity (Z DR ), correlation coefficient (ρ HV ) and specific differential phase (K DP ) from National Weather Service NEXRAD collected at Vance AFB facility at the first elevation angle from January 1st, 2015 to July 31th, 2019. The experimental results demonstrated that the CNN-based detector has better detection performance and measuring accuracy in range, velocity, azimuth and elevation and more robust to noise in comparison with traditional radar signal processing approaches and other state-of-the-art methods.Traditional fuzzy logic hydrometeor classification algorithm is a common way to classify precipitation type from dual polarization doppler radar. Then, the CNN-based model is evaluated on the dataset. Due to the lack of labeled radar complex data, we construct a radar echo dataset with multiple signal-to-noise ratio (SNR) for RTD. The proposed detection method exploits time and frequency information simultaneously therefore, the target can be detected and located in multi-dimensional space of range, velocity, azimuth and elevation. In this paper, we propose a model for multitask target detection based on convolutional neural network (CNN), which works directly with radar echo data and eliminates the need for time-consuming radar signal processing. Although radar signal processing has been revolutionized since the introduction of deep learning, applying deep learning in RTD is considered as a novel concept. Radar target detection (RTD) is one of the most significant techniques in radar systems, which has been widely used in the field of military and civilian.
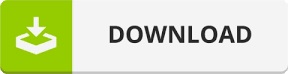